Tree mapping and reconstruction from aerial and terrestrial LiDAR data through topology-based techniques
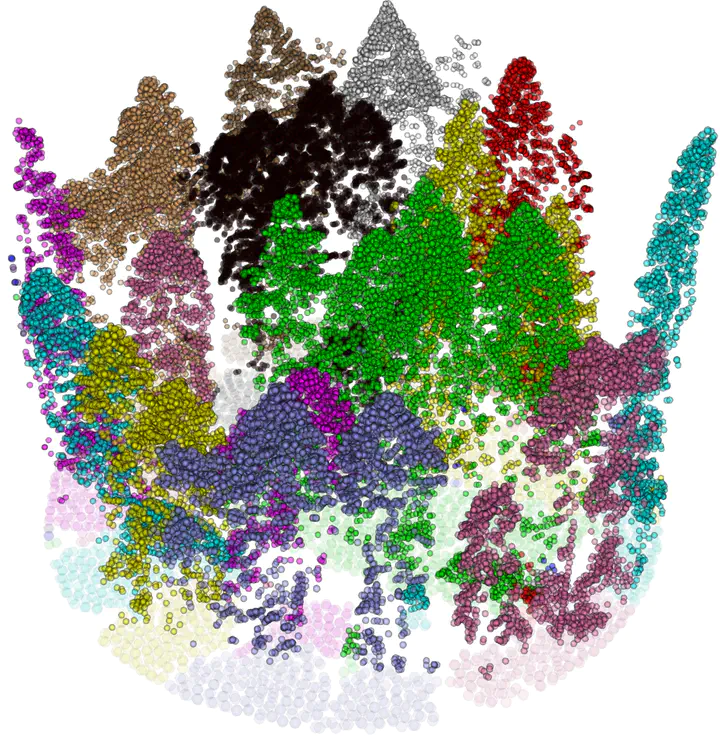
Light Detection and Ranging (LiDAR) techniques have dramatically enhanced our ability to characterize forest structures remotely by acquiring 3D point cloud samplings of forest shapes. Extracting individual trees from the forests plays a critical role in the automated processing pipeline of forest point cloud analysis. However, there is still a lack of automated, efficient, and easy-to-use approaches available to identify and extract individual trees in a forest point cloud. This is mainly due to inconsistent point cloud quality, diverse forest structure, and complicated plant morphology. Most existing methods require intensive parameter tuning, time-consuming user interactions, and external information (i.e., allometric function). In this reserach, we consider the problem of extracting single-tree point clouds from input forest point clouds. We propose two novel Topology-based Tree Segmentation (TTS) approaches, namely TTS-ALS and TTS-TLS, for airborne and terrestrial laser scanning data analysis, respectively. TTS algorithms are plug-and-play by nature and controlled by at most one parameter, ensuring user-friendliness. The implemented TTS software tools can extract single trees from 3D point clouds on various forest types, including conifer trees, broadleaf deciduous forests, and evergreen subtropical trees. By moving from single-time LiDAR point cloud data to multi-date point clouds, which are scanned from the same forest at different times, we will also segment and analyze LiDAR point clouds over-time.
https://www.sciencedirect.com/science/article/pii/S1569843222003338